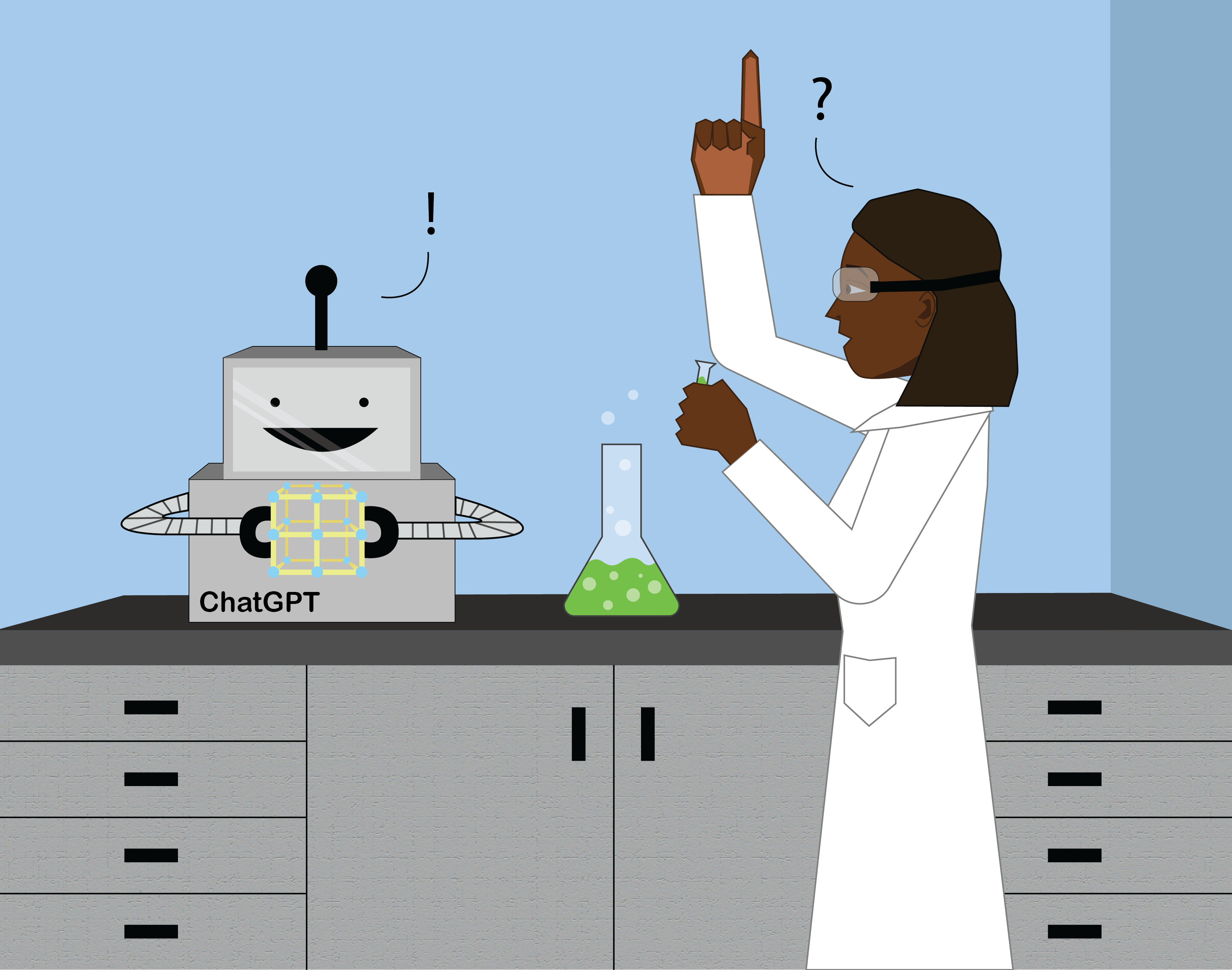
Harnessing Chatbots as Chemistry Research Assistants
WRITTEN BY NEIL LIU
ILLUSTRATED BY MANAL VISHNOI
Grappling with large amounts of experimental data can be burdensome for researchers. For decades, researchers have been developing new algorithms and training artificial intelligence (AI) models to shoulder such laborious tasks. With the breakthrough in large language models, including ChatGPT, a new revolution in chemistry research might have arrived. In a recent study in the Journal of American Chemistry Society, a team from UC Berkeley trained ChatGPT for a time-consuming task—searching academic literature. To further demonstrate the potential of ChatGPT, the team used this large language model to help them code a machine-learning model that predicts experimental outcomes and a customized chemistry chatbot.
The protagonist, ChatGPT, is a natural language processing model designed by OpenAI to generate human-like text based on a given prompt or conversation. Since its launch in August 2022, users have been inspired to discover numerous uses for this model, including using ChatGPT to create slam poems, make travel plans, and even simulate an entire chat room. ChatGPT also allows users to build their customized AI assistants within their own application through ChatGPT API, or application programming interface. As ChatGPT gained enormous popularity, chemists wished to harness the power of ChatGPT to mine and process valuable information from vast amounts of literature. For non-programmers, ChatGPT offered a better alternative compared to previous generations of specialized language models due to its less demand on users’ coding expertise and literacy in chemistry nomenclatures and reactions.1
Upon seeing ChatGPT’s potential in chemistry research, Dr. Omar Yaghi and his colleagues at UC Berkeley were eager to apply it in their research of metal-organic frameworks (MOFs). MOFs are highly porous, crystalline materials that consist of an array of positively charged metal nodes binding to the arms of organic “linker” molecules.2 Their repeating cage-like structures give them an enormous surface area, which attracts great interest from the research community to explore their usage in gas storage, catalysis, and much more. So far, over 90,000 different MOFs have been reported, which makes searching for synthesis conditions extremely challenging.3 Whenever researchers want to synthesize a specific MOF compound, they have to sort through hundreds of papers to look for synthesis conditions.

Figure 1. An illustration of the metal-organic framework inspired by Sergio Carrasco4 and designed by Rosalynn Mananghac.
Fortunately, in the paper titled “ChatGPT Chemistry Assistant for Text Mining and the Prediction of MOF Synthesis”, Yaghi and his team designed a workflow to turn ChatGPT into a more specialized AI assistant for MOF research, which they termed as ChatGPT Chemistry Assistant.1 When tested on 228 papers, the system extracted over 26,000 parameters relevant to the synthesis of approximately 800 MOFs with an average processing time of 56 seconds per paper. ChatGPT Chemistry Assistant also predicted MOF crystallization outcomes with over 87% accuracy.1 Remarkably, the Yaghi Lab constructed this AI assistant mostly with narrative language fed to ChatGPT, which leads to the question: how did they make an AI system accurate and efficient through natural language?
The answer was prompt engineering—carefully designing the prompts for the chatbot to steer ChatGPT toward generating precise and pertinent information. One problem the team faced was hallucination, a phenomenon where ChatGPT and other language models fabricate unreliable and misleading responses when the prompt is unclear or asking beyond the database. To address this, the team designed detailed instructions to make ChatGPT less likely to provide incorrect information. For example, the team made an additional prompt following their inquiry: “If you are uncertain, reply with ‘I do not know’”, and therefore forced the AI to answer based on its knowledge. The team also prompted ChatGPT to generate outputs in a table with fixed headers, making data easier to process by computer programs. Together, these two measures were important to the development of ChatGPT Chemistry Assistant.
After finding the proper way to instruct ChatGPT, the Yaghi Lab trained it for its first task – text-mining from academic literature. After several improvements, the team devised a text-mining method that worked in the same fashion as students read SAT articles. First, it filtered irrelevant paper sections such as references, then looked for paragraphs that contained explicit synthesis conditions, and finally summarized synthesis conditions in a table. Rather than relying on time-consuming conversations with web-based ChatGPT, researchers had ChatGPT create Python scripts to execute the text-mining on ChatGPT’s application programming interface. Conveniently, researchers only needed to specify requirements such as inputs and desired outputs in natural language, and appropriate Python scripts were generated by ChatGPT. In the end, the team was able to collect MOF synthesis data from hundreds of papers, which founded the basis for the development of a MOF synthesis prediction model and a MOF chatbot.1
Next, the team was ambitious to use the MOF synthesis dataset to train a machine-learning model to predict the outcome of the MOF crystallization to be either single-crystal or polycrystalline. Crystal lattices are distributed uniformly in single-crystal materials, whereas they form uniform grains separated by boundaries in polycrystalline materials just like a puzzle.5 Since single-crystal material and polycrystalline material have notable differences in physical and electronic properties, it’s important to predict the outcome of MOF crystallization when synthesizing specific materials.
During the synthesis of MOFs, metal ions are entrenched by their coordination with organic molecules, and many variables ranging from temperatures to types of solvents can affect the outcome.6 To make the model more efficient, the researchers predetermined the six sets of relevant factors based on experimental experience, namely metal nodes, linkers, modulators, solvents, their stoichiometric ratios, and reaction conditions, to create the training dataset. Then the team trained a classifier model using Scikit-Learn’s RandomForestClassifier, which combined the output of multiple decision trees to reach the most precise prediction. This prediction model could accurately predict and categorize the synthesis product as single crystal or polycrystalline.
Having utilized data-mining techniques to obtain MOF synthesis data, the team wanted to leverage this resource to build a MOF synthesis Q&A chatbot, making the dataset more accessible. With the help of ChatGPT, the Yaghi team compiled a large dataset including bibliographic information of each paper and MOF synthesis factors, such as reaction time, temperature, type of metal ions, etc. To be manageable by the language model, this information was converted to a specific format called text-embedding, which uses numeric vectors to represent semantic meanings: the closer two vectors are, the more similarity two sentences/words will have. Similar to the development of the text-mining process, the programming for text-embedding was done by ChatGPT. The resulting MOF chatbot could construct its answer centered around given synthesis information. For newcomers to MOF research, this chatbot could provide comprehensible data, reliable sources, and detailed explanations to make the learning process of MOF synthesis more efficient.
This study demonstrated the great potential of language models in the realm of chemistry. The impact of AI in chemistry transcends the boundaries of MOF research. Chemists, even those not familiar with coding, can set up specialized AI research assistants, potentially reducing the time consumed by routine work. Chatbot assistants can also make education more efficient if used correctly and fairly. For college students, having chatbots specialized in chemistry will not only offer learning options to strengthen our understanding outside of the lecture but also open the door to numerous fields of research.
References
- Zheng, Z.; Zhang, O.; Borgs, C.; Chayes, J. T.; Yaghi, O. M. ChatGPT Chemistry Assistant for Text Mining and the Prediction of MOF Synthesis. J. Am. Chem. Soc. 2023, 145 (32), 18048–18062. https://doi.org/10.1021/jacs.3c05819.
- Zhou, H.-C.; Long, J. R.; Yaghi, O. M. Introduction to Metal–Organic Frameworks. Chem. Rev. 2012, 112 (2), 673–674. https://doi.org/10.1021/cr300014x.
- Berger, M. What Is a MOF (Metal Organic Framework)? https://www.nanowerk.com/mof-metal-organic-framework.php.
- Carrasco, S. Metal-Organic Frameworks for the Development of Biosensors: A Current Overview. Biosensors 2018, 8 (4), 92. https://doi.org/10.3390/bios8040092.
- Holmes, D.; Bridges, A. Atomic Scale Structure of Materials. https://www.doitpoms.ac.uk/tlplib/atomic-scale-structure/printall.php.
- Stock, N.; Biswas, S. Synthesis of Metal-Organic Frameworks (MOFs): Routes to Various MOF Topologies, Morphologies, and Composites. Chem. Rev. 2012, 112 (2), 933–969. https://doi.org/10.1021/cr200304e.